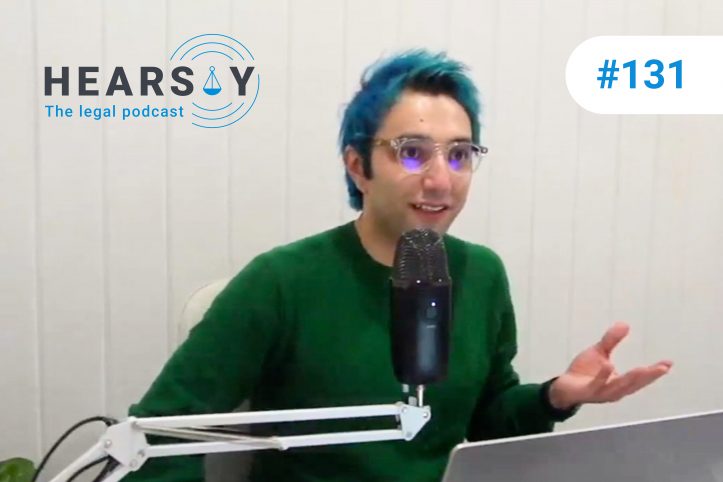
Want to listen to the full episode and all our other episodes?
Hearsay allows you to fulfill your legal CPD requirements every year.
Our yearly subscription is only $299/year.
With a yearly subscription, you can access all of our episodes AND every episode we release over the next year.
The Lawyer’s Guide to Generative AI: Where It Fits (and Doesn’t) in Legal Practice
What area(s) of law does this episode consider? | Generative AI; large language models; AI in legal practice. |
Why is this topic relevant? | In recent years, the legal profession has witnessed significant advancements in technology, with generative AI being one of the most transformative. As AI systems become increasingly sophisticated, they offer new tools for lawyers to streamline tasks, improve efficiency, and even provide new insights in legal research and document drafting. In early 2024, LexisNexis conducted a survey with over 500 lawyers between Australia and New Zealand, and 1 in 2 respondents reported that they were already using generative AI tools in their day-to-day operational tasks. Further, a 2023 report by McKinsey Digital estimated that today’s AI technologies could automate tasks which presently occupy up to 60-70% of employees’ time around the globe. Goldman Sachs released a similar report but specific to the legal profession, though its findings, that up to half of all lawyers tasks could be automated by AI, were the subject of some debate. However, the appropriate and effective use of generative AI in legal practice remains a complex and evolving issue. Misunderstanding AI’s capabilities and limitations can lead to ethical challenges, errors in legal work, and potential negligence. As such, there are important questions to be asked about when it is, and is not, appropriate to rely on AI in legal practice. |
What are the main points? |
|
What are the practical takeaways? |
|
Show notes | Alimardani, A. (2024) ‘Generative artificial intelligence vs. law students: an empirical study on criminal law exam performance’, Law, Innovation and Technology ICLR Conference Paper (2024) ‘The Reversal Curse: LLMs Trained On “A Is B” Fail To Learn “B Is A”’ |
DT = David Turner; AA = Armin Alimardani
00:00:00 | DT: | Hello and welcome to Hearsay the Legal Podcast, a CPD podcast that allows Australian lawyers to earn their CPD points on-the-go and at a time that suits them. I’m your host, David Turner. Hearsay the Legal Podcast is proudly supported by Lext Australia. Lext’s mission is to improve user experiences in the law and legal services, and here’s how the legal podcast is how we’re improving the experience of CPD. In recent years, the legal profession has witnessed significant advancements in legal technology and since November, 2022, generative AI has been perhaps the most transformative. As AI systems become increasingly sophisticated, they offer new tools for lawyers to streamline tasks, improve efficiency, and even provide new insights in legal research, document drafting, and document review. In early 2024, LexisNexis conducted a survey with over 500 lawyers in Australia and New Zealand, and one in two respondents – half of all respondents – reported that they were already using generative AI tools in their day to day operational tasks at work. Further, a 2023 report by McKinsey estimated that today’s AI technologies could automate tasks which presently occupy up to 60-70% of employees’ time around the globe. Goldman Sachs released a similar report but specific to the legal profession, though its findings that up to half of all lawyers tasks could be automated by AI were the subject of some debate. However, the appropriate and effective use of generative AI in legal practice remains a complex and evolving issue. Misunderstanding AI’s capabilities and limitations can lead to ethical challenges, errors in legal work and potential malpractice. As such, there’s important questions to be asked about when it is and isn’t appropriate to rely on AI in legal practice. Now we’re joined today in the recording room by Dr. Armin Alimardani, lecturer at the University of Wollongong School of Law, whose research focuses on the intersection of law and emerging technologies, including AI. As an AI expert – is it okay if I call you an AI expert, Armin? Armin has extensive experience in examining the ethical and legal implications of AI and is well versed in the challenges and opportunities that AI presents to the legal profession. Armin, thank you so much for joining me on Hearsay. |
00:02:14 | AA: | Well, thanks for having me. |
00:02:15 | DT: | Now, before we get into it, tell us a little bit about your background in academia, in research, in law… How did you get to studying this topic? |
00:02:21 | AA: | Well, it might be a bit surprising for everyone because I didn’t like law, so I was looking for a way to get away and then I discovered criminology as one of our subjects during the undergrad and then I was like, “okay, you can connect law to many other things, including sciences and technology,” and that’s how I became interested in law again and after that, I was just pursuing the intersection of law and technology. And I did my Masters on genetics and crime and criminal behaviour, my PhD on neuroscience and crime and criminal behaviour, and how they use this type of evidence in New South Wales courts. And then in 2018, around that, I became familiar with OpenAI and I did a couple of projects here and there in different universities on AI and by 2022, we were just making our own first AI powered educational and research chatbots. |
00:03:16 | DT: | So you studied law, didn’t really find a passion for it in the black letter law, but found a newfound passion for it in the way it intersects with technology, and I suppose AI has been since 2018, one of your key focus areas. |
00:03:30 | AA: | Yeah, exactly. |
00:03:31 | DT: | All right, well, let’s get into it. We’re talking about generative AI today. Now, it’s useful when we talk about AI to define some of these terms, right, because they’re not always terms that are easily defined, or even that there’s a consensus about their definition but we’re talking about generative AI as opposed to AI. Let’s start at the broadest and drill down. Armin, if you had to define artificial intelligence, let’s define that first and then follow that on for me with a definition of what subset of that is generative AI. |
00:04:03 | AA: | There are many ways to define artificial intelligence. One is, let’s say, algorithms or robots or bots that can mimic human cognition, do the thinking that we do, do the analysis that we do. And we have older school AIs that you say “if that happened, do this. If this happened, do that.” They’re very simple and they’re not that effective but then machine learning happened; that is, we give a lot of data to the machine and say, “we’re not going to tell you what the rules are, we’re going to tell you this is how things happen, you figure out the rules.” And after that, whatever data we give it based on the rules that it has discovered, it can address any new problem. So that’s the machine learning, and generative AI is kind of a subset of machine learning, that we give a machine a lot of data to figure out the correlation between words or, it’s actually tokens but let’s say words to simplify it, and to generate a new word based on the training data. So it’s not about copy-pasting something. It’s about generating something new. |
00:05:07 | DT: | Yeah. So I suppose what you referred to there in terms of your example of generative AI, what we call a “large language model” that predicts tokens. And you mentioned tokens, which are whole words or parts of words, I suppose. So a short word like ‘the’ might be a single token, a word like ‘antidisestablishmentarianism’ might be 10, and a large language model is, as you said, a next token predictor, its role is to make an inference about the next token in a sequence of tokens based on its training data, based on the prevalence of token sequences in the billions and billions of words that it has been exposed to in the training epochs it’s conducted. Large language models are probably the most relevant form of generative AI for lawyers because so much of the work we do is bound up in text, right, cases and contracts and legislation… Large language models have both the greatest promise for us and the most exposure to the kind of material that we work with but are there other kinds of generative AI that we should be thinking about? |
00:06:06 | AA: | Oh, we have image generators, sound generators, movie generators, and there are some new ideas about this and there is this institution that they use generative AI to generate images of the asylums in Australia to, for the first time, depict how it looks and how it feels to be in there because so far it’s been only words and we were never able to see it and now there are discussions about recreating the crime scene or through generative AI creating videos that shows what happened at the time of crime, those kinds of things but usually these come with problems rather than helping the legal profession. Of course, they could be deepfaked as evidence, and it would be very difficult to sometimes distinguish deepfake from the real image. |
00:06:50 | DT: | Yeah, you can absolutely imagine some of these other models, like diffusion models, that create images being used to fake evidence. I’m thinking of that, not an image model, but a deepfake voice of president Joe Biden during the democratic primaries earlier in the year, encouraging people not to vote, which is a great example of how some of this technology can be misused and I could easily imagine image based models being used to create some very persuasive but unreliable, not very probative evidence for courts to deal with. |
00:07:22 | AA: | I can imagine there are going to be many cases in court soon for deepfakes with the new legislation criminalising sharing deepfake material and because it’s so accessible, many people are going to do it anyway, and they will get caught at some point and yeah, I assume there are going to be many cases like that. |
00:07:40 | DT: | Yeah but for a lot of our listeners, and the topic we’re talking about today is when it’s appropriate for our listeners, lawyers, legal practitioners to use generative AI in practice. We are going to be talking about large language models, really. How can large language models help us to understand the contents of legal documents and cases, how can they assist us to draft legal documents to better refine our writing, all these sorts of things. And I suppose one topic that’s really interesting to me is, as we described before, a large language model is a next token predictor, it’s designed to predict to the next token word or part of a word in a sequence, but we have other prediction models like the one on your phone where you type a part of a message into your messaging app. It might suggest some words, but plainly doesn’t have the same capability as a large language model. Pretty quickly, if you were to just keep selecting the suggested word in your messaging app, you’d have some nonsense. There’s a bit more going on under the hood with a large language model. Tell us a little bit about how they work, in particular, what we call the attention mechanism. |
00:08:43 | AA: | Yeah, so that’s a great question and many have asked why should we even learn the underlying mechanism, even though I’m going to explain it in simple terms but for me at least, it’s been very much about understanding the limitations and capabilities of generative AI, instead of reading some of the nonsense I found on social media and other places about generative AI. Many academics also say that you’ve got to learn the mechanism if you want to use generative AI. So, one thing is it’s pre-trained, which means they feed a lot of data on the internet, but the most important thing is, it’s not all the data on the internet. So there are gaps in the data but it learns from the internet data and it finds correlation between words, like which words come close to each other a lot. So when I say ‘legal’, what are the words that come after ‘legal’ often? And if it’s ‘analysis’, for instance, it makes it more likely when it’s predicting the next word, ‘analysis’ comes after ‘legal’, but you can imagine in various contexts, sometimes like in Australian context, maybe ‘analysis’ comes after ‘legal’ a lot because that’s how we use it but in the US it’s a different term. So if they fit the data from the US servers, we would get very wrong answers, and that’s one of the ways that hallucination may happen and sometimes the questions are about things that weren’t in the dataset that we provided for the training. So, because it’s trying to generate the next token or word anyway, it’s not about generating truth or lie or hallucinating, it’s about generating the next token, it would give us things that are not real or we know some people say “white lies” – it’s not lying, it’s just generating the next word as it’s being designed to do that. |
00:10:22 | DT: | Yeah. I mean, you have been researching this a lot longer than most people have even been aware it existed, pre-trained transformers or large language models. You might remember the model card for GPT-2 that explicitly said “because large language models cannot tell truth from fiction, we do not recommend the use of GPT-2 for any use case that requires the output to be true.” |
00:10:42 | AA: | Yeah. |
00:10:42 | DT: | That still applies. |
00:10:43 | AA: | Yeah, and back then they thought GPT-2 was so huge that they didn’t even want to release it to the public. And anyway, it came to GPT-3 and that was the model I learned a lot from but, yes, knowing that it relies on data and what type of data it’s being fed, like whether it’s legal data or jurisdictional, it can really impact how it gives us the next answer but one of the things that is usually misunderstood about language models is that it doesn’t predict the most probable word. It predicts a series of most probable words. Technically it predicts all the probability of all the words that it has in its dictionary, like its 50,000 words, something like that, and then from the top ones it selects more randomly and that’s the reason every time you use it with the same prompt, you’re getting different answers because there’s a randomness there and it gives you different outputs. It’s like domino, if you’re giving it one different word at the very beginning of the sentence, the rest of it could be different because all the probabilities change and what is important, considering that for lawyers, is that in law, we have very specific terminology we use in various contexts, and for the public you can use a synonym, but in law you cannot because it would mean completely something else or nonsense even. So that randomness can really impact how we use it in law, and we should be very careful about the terms that are being selected by the language models because of randomness, and it might use one of the less probable words that doesn’t fit that context. |
00:12:13 | DT: | Yeah. I suppose the other thing to think about with that is, the prompt, the instructions that you provide to the model, that is the starting off point for that predictive task and so the selection of tokens, the selection of words that you input into a chat based model, ChatGPT, or an instruction based model, is going to influence the output dramatically. In a legal context, a synonym could result in a completely different answer. |
00:12:36 | AA: | Yeah, yeah, exactly. So, if in your prompt, you don’t use proper terminology, you may not get proper terminology as output. So yeah, it’s very important how you frame it. And that also means when we say next word prediction, it’s not relying on the last word in the sentence, it’s relying on anything behind it. So it could be 100 words or 1000 words, and it makes a comparison in terms of the correlations between all these words in the dataset that it was trained on. So you can imagine a lot of these thin strings are being attached between all these words and they’re pulling each other to determine what’s the next possible word. So, the more words you have, the more calculation it needs to do to predict the next one and every time the next word comes in, then it does the whole thing again, then make a calculation between all the words. And again, for lawyers, it means that the lengthier your document is, the more likely the output you’re getting is not the output you want. You’re making that calculation very complex to the point that he may not be able to find what are the best, most probable words to put after. |
00:13:24 | DT: | I love that analogy of the strings pulling taut in different directions. I love that. So, Armin, you made a couple of great points there. The first is that there’s a great amount of misinformation available about how these models work. I can remember in late 2022, and really it hasn’t gotten any better, the level of misinformation about the way a large language model, quote unquote, “accessed information,” people thought that you could, although this is now available with some other tools, you could enter URLs and have the model read the website. And I think it’s important to understand that this next token prediction task is being done after the training step in the sense that the training material, as you said, some content, a lot of content from the public web, but not all of it, is used to train the model but the model works like a human brain, doesn’t it? It’s made up of neurons, made up of nodes, billions of parameters, and the training data ends up being represented in numerical values, what we call weights and biases on each of these nodes but when the model is answering a question for you, it’s not looking up the information it’s been provided with. It’s not going, “oh, I think there’s a good article by Mallesons” or “there’s a good article by Freehills about that topic.” It’s performing this task that, as you said, we don’t really, with any exactness, know how it does so, but it’s performing an inferential task based on those weights and biases, all of the settings in its billions of parameters, not accessing a particular piece of information. And so the material that is relevant to your question about Australian law is being influenced just as much as it is about other training data about US law and UK law and all of the material that it’s ingested in the course of the training process. |
00:15:25 | AA: | Yeah, exactly and that’s, I think, one of the very important things – that it creates this neural network, it’s not like an ocean of data when we ask questions. Some say that’s one of the limitations. It should be like that. It should be for the language model to be able to access data. But again, as you said, it’s so much like humans, when you read something, it’s not that somebody asks us a question about what we read, we just read it back to them. It just comes through our brain and the connection between the neurons, and it’s not like data is somewhere in our brain, we go and find it. So it has that kind of similarity and again, that’s one of the reasons it makes it less accurate because it’s relying on memories and connection between the words, not the actual word that is out there. |
00:16:06 | DT: | Yeah, absolutely, and I suppose there are other design paradigms for AI applications that produce that sort of result, that kind of looking up of information rather than the parametric approach, what we call ‘Retrieval Augmented Generation’ is one approach to that, where you connect a large language model to a source of static data and use various search methods to retrieve relevant information that the model uses as a kind of ground truth or input. |
00:16:35 | AA: | Yeah, yeah, so this is becoming very popular recently, I noticed and has been discovered by many companies that, “oh, this is something we can do.” And the interesting thing is that it’s not relying on language model necessarily, or ChatGPT or whatever language model you’re using. We are using a different system called embedding and that’s one of the reasons that can potentially make it more reliable, even if you have a 100 page document because it finds the relevant information. Let’s say it finds two paragraphs that are relevant to your question. It brings those two paragraphs to the language model and says, “now read these two paragraphs, answer that question.” It’s not the language model that reads the 100 pages of the text and it works much better than relying only on the language model. |
00:17:19 | DT: | Yeah, one of Lext’s products called Playbooks uses a RAG approach to performing the document review tasks that it does, and the way I sometimes explain that is, you know, it’s the difference between as a lawyer, if a client asks you a question, trying to answer based on your memory of that topic from law school, or saying, “let me check and get back to you” and going and looking at the legislation and case law first and then answering. |
00:17:41 | AA: | Or to put it better, you will ask your assistant to go and find the relevant information and take a copy and they say “here is the three relevant pages” and they don’t summarise or anything, they give you the exact text but out of many books or whatever you have they said “here’s the information, the part relevant.” And you read it and yet you’re not relying on your memory, you’re relying on the actual text in front of you. |
00:18:03 | DT: | Exactly. And I guess what that does from a design perspective is it reframes the task for the large language model in that if you ask a legal problem question to a large language model alone, you’re asking it to perform an analysis task, a logical task, whereas if you pose that same question to a RAG based design, you’re giving it a reading comprehension task, right? You’re providing it with the external information with which to answer and then asking it to perform a reading comprehension task in order to answer, but that really brings us on to one of the hottest topics in large language model performance today and by today, I mean the 13th of September, because we’re talking about the capacity of large language models to think, as it were. Tell us a little bit about that. |
00:18:47 | AA: | Yeah, so, see, language models, and I don’t want to exaggerate something, I may sound like that, so giving you just a caution that may seem too high, but when it comes to language models, we hack at the system, because language in humans is one of the biggest features, because language help us not only communicating, we can have a record of things and pass it to generations and society progresses way faster because we already have the previous information experiences. And yeah, because language is so accessible to us humans, when you chat with a computer, it really feels like you’re interacting with other humans, especially the later models that are just getting better and that, right, and it feels like they’re thinking, but recent research, and I say recent, it goes back maybe to 2 to 6 months ago, showed that unfortunately, they’re not that good at thinking that they appear and here’s what it appears they do is just they memorise the data and because we feed them an ocean of data, whatever you ask, there’s something in the ocean of data that makes the connections and give you an answer with that and when it comes to reasoning, if you say “2×2, what is the answer?” It can come from the data, but if “2×4” is not in the data, let’s assume, it might memorise the chain of reasoning, like how 2×2 happened, it would do that to 2×4, and which is technically machine learning, you learn the rules, you extend it to other matters. But, if you go beyond that, something that it hasn’t memorised the chain of reasoning because it wasn’t data, it cannot go beyond that according to the experiments. Let’s assume liquid, we don’t have liquid on earth or any part of the world and suddenly today we discover, other than gas and solid, we have liquid as well and these are the features and ask the language model “based on the features, I throw my phone in the liquid, what happens?” It’s very likely it will fail because it requires original reasoning or type of creativity. And unfortunately it cannot do that and it gets even more confusing because there’s a paper called The Reversal Curse and one of the most disappointing aspects of language model is in that Reversal Curse paper, they ask who is Tom Cruise’s mother and I don’t know what’s their name, but let’s say Jane Doe and it says, “yeah, Jane Doe” and if you ask, “who is Jane Doe’s son,” it cannot tell you. That’s the ‘Reversal Curse’. This is such a simple reasoning that the model cannot do. If you provide the information, the text for it and say, “yeah, Tom Cruise’s mother is Jane Doe. Now, who is Jane Doe’s son?” It can do that but if it comes from that neural network, it cannot do the reverse. It gives us the idea of how limited are language models, but because the datasets are so big, it gives us the feeling that, “oh, they actually are reasoning or they can or they can do these kinds of things.” |
00:21:35 | DT: | Yeah. I mean, the old adage is that “what’s easy for machines is hard for humans and what’s hard for machines is easy for humans.” |
00:21:42 | AA: | That’s the Reversal Curse. |
00:21:45 | DT: | Exactly. So another example that I’ve seen today with the o1 model that we hinted at earlier, this classic test of reasoning capability is called the strawberry test, which is just to pose to a large language model; “how many R’s are in the word strawberry?” It’s a trivial task for a primary school aged human. “There are three R’s because we can just count them,” but there’s no material in the training data. Funnily enough, no one has written an article on how to count the number of letters in a word. It’s just so obvious. |
00:22:14 | AA: | Yeah or how many R’s in a strawberry because it technically memorised it. And I saw that video clip that they talk about it because that, “how many R’s are in strawberry” has been around for a few months and when you try it, it doesn’t give you the answer, usually it says “two” unless you prompt it really hard and properly and we do that and the reason it cannot do it is because they’re tokens. So it understands the words based on tokens or let’s say word size, not letter by letter and if no one in the, you know, data set that is being fed to the AI never mentioned it, it cannot do that type of reasoning. It doesn’t have the capability, but there’s o1 model that was released on 13th of September, which is today. They want to address this reasoning problem, like simple questions that it cannot answer. And I’m talking about very simple questions, but with the newer model, what they do is they’re giving the model more time to, what they say; “thinking”, and usually takes two seconds to give you the output, it takes 25 seconds to give the output. So it’s taking its time to give the output but what it really does, at least on the surface, if you look at it, is that it’s doing what we call a chain of reasoning. So we humans, usually, if we want to do something complex, we just don’t go and do it. We break it down into steps. First, I’m going to do this and we imagine that process and AI doesn’t have that imagination process or in the dataset, we don’t have that imagination process that we do in our head. When it comes to text, we write the output. We don’t write what we were imagining. So that was lacking and what they’re trying to do is they’re adding these smaller steps to break down the task to much smaller ones and even going back and checking whether the input and the outputs of these two are aligned and it takes more time. It means it’s more expensive for OpenAI to run it, but it has at least answered some of those questions, ridiculous questions or easy questions that he couldn’t answer before. And people are very hyped to see what else it can do. |
00:24:13 | DT: | Yeah because as you say, with the right methodologies, large language models can through their predictive task answer some of these questions. For example, if you were to say, even to an older model, “take the word strawberry, break it into a list comprised of each letter in the word, and then go down the list and count the number of times R is in it,” it might be able to answer that. |
00:24:34 | AA: | That works. I tried that. That’s a way to get it to do it. |
00:24:36 | DT: | Yeah because it turns it almost into a coding task, right? |
00:24:39 | AA: | Yeah. |
00:24:40 | DT: | It’s a methodology that it better understands, or rather, you give it a methodology at all, where there previously wasn’t any but this thinking capacity, as you described, is allowing the model an opportunity to devise some of its own plausible methodologies for answering some of these questions. |
00:24:58 | AA: | Yeah, and it’s a very human thing, this chain of thought, and chain of thought always helps us to get to the answers more accurately, and they’ve tried it before, forcing language models to outline various steps they should take to get to the answer, and they always work better. It’s just like if I ask you “2×2”, you immediately say “four,” you don’t think what is the process of that. So, so far again, I’m simplifying language models, it’s been doing that, they’re just spitting it out, but now it’s just sitting down and saying “2×2. It means we have a number two, that is 2+2, that is four.” So it’s doing the process now and it goes back and checks that process. And some parts of the process look ridiculous, the way it’s analysing it, it says, “I’m thinking about this and I’m analysing this, I’m looking whether this part is clear.” It’s very interesting, kind of human, but so far it’s working for some of the more difficult tasks. |
00:25:48 | DT: | Alright, let’s get on to how we apply this in legal practice. Some of our listeners might have been real early adopters and started to use ChatGPT as early as late 2022 to help with some of their work but certainly by today, late 2024, we have CoPilot available to many lawyers, ChatGPT embedded in many enterprise applications, other models embedded in enterprise applications, generative AI based point solutions for legal tasks, legal technology specific software. So, generative AI is really everywhere for the lawyer who wants to use it. What are some of the best use cases from your observation and experience for lawyers and people doing legal tasks? |
00:26:30 | AA: | With the new model, I don’t want to really tell you what it cannot do or what it can do and how good it can do because we haven’t tried the new model yet, but I usually don’t give advice to anyone and say, “oh, it’s good at this and that, go and try it.” I say, “go and try everything.” When I’m organising workshops for law firms, I never say, “okay, I’m going to come and do it.” I say, “I need an hour with you just to see what you do and how you’re doing things.” And eventually for every task you do, you can try ChatGPT or any other generative AI model, and sometimes you figure out that, “okay, there is a way. If I prompt it this way, if I do this and that, it would give me the output that is good enough or helping me,” and there are lots of them that don’t work. And it’s creativity, and creativity is risky because you end up wasting your time figuring it out but if as a firm, this is your policy that whoever found a good thing, come and share with us, you can imagine after a few months where it’s going to go. So a lot of it is about being persistent, knowing how I’m going to prompt this and how I’m going to be responsible about this and what are different ways of prompting. But, one of the most important things I always tell people is that whatever you’re using for, don’t use it as a knowledge database as we discussed. It’s not like Google. Use it as a reasoning engine. And with that, I always say it means provide the relevant information and ask it to do something with it. So you say, “here’s legal information. Here’s what I want you to do, and the tasks requires first; do this, do that.” It will do way better and in one of the experiments we had, it almost never hallucinated. Never. It was shocking, but it almost never hallucinated. So if it has the relevant information, it can do a way better job. |
00:28:15 | DT: | Yeah. I suppose that means one good example is something like legal document review because you’re providing the information and you’re providing a reading comprehension task. “Based on this document, tell me these things that I want to know about it.” |
00:28:30 | AA: | Yeah, exactly. So, when I say for anything – because when it comes to legal tasks, we always have some kind of document or we have this report we have to write and these are all things that they made language models to be capable to do, but not necessarily good enough and not necessary for law – and the reason I say you got to try every single one of it is that if I tell you GPT-4o is good for legal analysis, for contracts, the next model may not be as good. So again, in my research, we found out that some of these updated models weren’t performing as good as the previous model when it comes to very specific tasks. So again, my recommendation of using this for this purpose, putting me in the wrong position, and majority of us don’t know, but every few months, they are releasing a new version of the same model, like for GPT-4o, I think we have three models right now, but you don’t see it in the front end, you have to go in the back end to see the dates of the release and how they are different to each other. |
00:29:31 | DT: | Yeah, these are models which are architecturally almost identical, but they’re checkpointed at different points in time. |
00:29:38 | AA: | Yeah, exactly and that helps us to be able to compare because when they change GPT-4o and update it, they don’t touch those fixed models – “static models”, they call them. They just change their very latest one, this “fluid or continuous model”, I think they call it. So you can go and compare them and if the model, let’s say 13th of September, is working for you, you can happily use it and if there is an update on the model, you won’t be worried that “oh, it’s going to change the outcome” because you know that 13th of September is working and you’re still using that later on if you want you can go and check the newer one and see whether it works for your task. So, it’s not that one day suddenly everything changes for you and “oh my god, it’s not working anymore” because we have those static models, if you use the static models. |
00:30:25 | DT: | Yeah. Well, I remember when GPT-4o was released, we’re speaking to some other legal technology companies who said, “oh yeah, we switched over to GPT-4o straight away.” And I was shocked. I said “well, haven’t you tested to make sure that the model behaves as the previous one you were using does?”, and they were like “oh, but it’s just better” but of course we didn’t switch to GPT-4o for our generative AI powered applications until we had comprehensively tested that it was performing those tasks just as well as it had in the past, or if not better. So, you’re right, for a really specific use case, newer doesn’t always mean better. |
00:31:00 | AA: | Yeah, exactly, and they tweak them and they see what are the popular tasks people are doing and if it works for those tasks, then they release a model based on that. They know some aspects are not as good but interestingly, anyone can access these models I’m talking about like when I say they come with the date. It’s in the back end of OpenAI. When you log in to OpenAI you use the same username and password as your ChatGPT, it will let you in, and you can see all these models – there are like 15-20 Models there – and there are more settings that you can change to get different outputs. Yeah, that’s something that’s hidden, but it’s very accessible. I always recommend lawyers use the back end because there are many more options there. |
00:31:41 | DT: | Yeah, without asking you to generalise then, can you give us an example of maybe one really good use case or great use of generative AI that you’ve seen in your work with law firms? And then maybe just to contrast, a use that maybe wasn’t as good. |
00:31:54 | AA: | Yeah. Alright, that’s a good question. So there are many different uses some law firms are doing, and we’ll get back to that, but one of the most popular ones is contract writing and I noticed many law firms gave it a try and they figure out what type of information they need to provide and they learned that if you give it a template and then the variables it would understand how it’s supposed to change things but two law firms that I kind of was in contact with, they were doing this in very different ways, like the prompting was really good from both sides, but one law firm had this policy of verifying the output by two other people and the other law firm didn’t have that policy and the person who was generating it wasn’t really verifying it that much because they had this confidence in the output of the generative AI that it looks good and it works really good, and that’s one of the biggest worries for me, over-reliance. And it’s nothing that people don’t know. People actually, when you tell them, they’re still not doing that verification at the end and I again did it in a study last year with my students and I taught them how to use generative AI and there was this assessment and say “okay go and use it. This is a responsible way of using it, and reflect on how you use it.” I could not believe how many of them, they just copied the output without verifying. I was like, “what did we do with the five sessions, every time I told you, you’ve got to verify that?” And it’s a serious problem. Even Microsoft has raised it, that they are worried. Even OpenAI has raised it and they say, “as the models get better, we trust them more. And it makes it less likely we verify the output properly.” And even I missed verifying twice because I was like “the task is so simple” and later on I realised it didn’t do the proper job. It was just “generate 10 random numbers” or something like that, but I realised the numbers were in sequence so instead of being random, and I didn’t check it and I was almost in trouble before figuring it out, so I over-relied. Like I was like “the task is easy or it has done it before,” something like that. It happens so easily that it’s one of the biggest worries right now that people are going to, yeah, rely on it and it turned out, even if you teach people, it wouldn’t work the way you may think. Other than my study, there was a Stanford study in the business school, if I’m not wrong, and in one of the tests, not all of them, people who use generative AI, they perform worse than those who didn’t use generative AI and those who were trained how to use it, they perform even worse than those who weren’t trained how to use it. So it works somehow in reverse and it shows like the more you know it, it can give you more confidence that you’re able to identify the problems or verify or push yourself to improve it other than saying that, “oh, the output is good enough, I think. So I’m not going to do anything else with it.” |
00:34:43 | DT: | Yeah, I think that need to verify is super important and I suppose it suggests that when using it for a task like contract review or any kind of, as we were talking about before, Retrieval Augmented Generation based task where you’re asking the large language model to look at a larger corpus of data and give you answers based on it, it’s really important to be using a tool or an application that makes that verification process easy, right, there’s RAG based tools that can give you the referencing for where it’s found that information. |
00:35:13 | AA: | Yeah, yeah. I was just in a session yesterday at the LexisNexis AI focus group and talking about their products and asking our opinion and what I noticed was that they were trying to address a lot of worries that we have about verifying the output and when you ask about a case or information, it’s relying on multiple cases and verifying that it would be time consuming. You’ve got to find the cases first. You’ve got to find where it found the information and then paraphrase it for you. It’s really time consuming. So what they have done, using like RAG systems, is that it tells you exactly the paragraphs that it has taken the information, and it’s right in front of you, with one click, you can see how it works. So there are approaches that help to verify. The question is, would people still verify that, even if it’s right in front of you? |
00:36:01 | DT: | Yeah. One complaint I’ve heard from media organisations about Perplexity, the kind of AI powered search engine that provides summaries with footnoted sentences from where it’s found in information, is that the footnotes are so small and so out of the way that very few people really click those links to verify the information or read more. Perplexity says that that’s not true, that they make up a large source of the traffic for these large newspaper and publishing organisations that make that very complaint, but it is symbolic of this problem of you have to verify and sometimes even being given a very convenient way to do that, a user doesn’t necessarily do so. But I suppose as lawyers, when we’re thinking about the right use cases for our professional work, I might put it as highly as saying we have an ethical obligation to verify those answers and maybe by framing it as an ethical obligation, we’re more likely to do it. |
00:36:57 | AA: | Yeah, and I think it somehow implies that law firms that want to get into generative AI, it’s not that I’m going to instruct people to go and use it and try it. It goes way beyond that because people don’t know what is the responsible way of using it, or if they know, they may not follow the instructions the way that it’s supposed to, and you might need to have multiple activities throughout weeks and months to force people to do the verification at the end to make it some kind of a habit; if you don’t do it, you feel like something is missing. And this kind of process is not in the literature, it’s just coming out and we are learning that, but it’s not that simple and I heard a lot, even at universities, they say, “let’s just tell the students to use them,” but it’s not that simple. It has a lot of psychology in it. |
00:37:41 | DT: | Yeah, absolutely. Our discussion about comparing the performance of different models for different use cases has reminded me of something we were talking about just before we started recording, which is the challenge in measuring performance in any kind of scientific way. Tell us a little bit about the benchmarking that we have available for large language models, or I guess more accurately, the absence of any benchmarking that we have for legal tasks. |
00:38:03 | AA: | Yeah, so it has become one of my favourite topics recently; benchmarking. So any language model that comes out, they usually put a table of the various benchmarks that they compare their language model to other language models because they’re using the same benchmarks and some of them are like 12,000 questions and they’re pretty lengthy but recently, again, it is only mostly in the last six months to one year, that it turned out many of these benchmarks are not reliable to be used for AI, maybe for humans, but not for AI because of – let me give you a couple of examples. One is that if you change the order of choices, it would change the outcome. Some of them have a bias to choosing the third option. And they get the answer right, but if you just change the order of choices, it would get it wrong. |
00:38:50 | DT: | There’s a correlation there, but not a causative link. |
00:38:53 | AA: | Yeah, exactly. That’s bad fine tuning from the AI companies. The other is, if you add space for the choices, it would change how they answer questions, but even worse, is that they figured many of these benchmarks, they didn’t copy the questions properly. Some of them, they have no choices. It’s just a question without any choice. So the model just gives you a random A, B, C, D without knowing what they’re even choosing, if that makes sense. |
00:39:19 | DT: | Yeah. It’s just being presented with a multiple choice question, and the next plausible token is one of; A, B, C or D, irrespective of what the answers are. |
00:39:29 | AA: | Yeah exactly, and sometimes the questions are wrong they realise, but the most important thing is just changing the order, it would impact and sometimes 20% drop but when it comes to reasoning again, you want to know whether AI can reason. How would you do that? You would change the numbers in a question. Like it says “there are two apples, two oranges and blah, blah, blah.” Instead you say “three apples, three oranges” to see whether you can do the reasoning. And it fails in many cases. It goes, again, 30% reduction in the benchmark performance. And so benchmarks are not the best things to test these things and the other is that they’re not reliable and also they show AI models don’t do reasoning properly and many of these benchmarks, again, have contaminated data, which means the questions are outside. They were part of the training data of the AI. So they memorise the answers without knowing what they’re doing. But, the most important thing, as you mentioned, as far as I know, we don’t have a proper benchmark for law. And the reason we don’t have it, I think, is creating benchmarks, is hell of a work. You might think, “okay, I’m going to generate 100 questions.” It’s definitely not enough. You’re going to need way more and the question is how many, and what are you measuring? What aspects of legal analysis and whether these questions are standard and would actually assess the legal analysis and this one is going to assess the knowledge of the model and how many people do you have to draft the questions? How many do you have to verify the questions? And keeping these questions off the internet so they don’t become part of the data set of future models is also very difficult to do. |
00:41:02 | DT: | Yeah, that’s a particular problem with what you see in a lot of marketing copy for AI companies who are promoting the capabilities of their models for legal use cases is that they’ll comment on its performance on a bar exam but the problems are kind of everything you’ve just described there – the bar exam might not be made up of enough questions to really be a comprehensive benchmark, the bar exam questions might be available in the training data so it’s fit to those questions already, and then what we also see is they’re misreported. So a company might report that the model performed in the top 10% for this bar exam, but it only performed that way for the multiple choice section and it did absolutely atrociously on the essay part. So, a human bar exam designed to test the readiness and aptitude of a human being to be admitted to practice as a lawyer is not really an appropriate benchmark to test the capability of an AI for legal tasks. |
00:42:00 | AA: | You raise a very good point. I totally forgot. So my most recent paper that I published is about that; GPT-4 being better than 90% of US bar exam test takers. And I was like, “it really is not that good.” So what I did – I tried it actually. I got AI generated text for the criminal law exam last year at the end of the year, I wrote them, hand wrote them in exam booklets, I put them between student exam booklet answers and I gave them to my tutors to mark them, so they didn’t know they’re AI papers, they thought these are all end of the year exam papers for students. And they marked them and the results were a disaster. So five of them I didn’t prompt engineer. Three of them failed. Two of them passed with 31 and 33 out of 60, 50-55%. |
00:42:43 | DT: | Scraping in. Yeah. |
00:42:45 | AA: | Yeah, and those that they prompt engineered, only two of them were much better and they were like 60-70%. And yeah, none of them reached 90% better than the students on average when I compared them. So it’s very important where you’re relying on these benchmarks and which benchmark you want to use for your work. |
00:43:03 | DT: | So knowing these problems with benchmarks, that they’re either not specific to law, they might be affected by biases, there might not necessarily be a causative link between the result and the model performance, all of these problems and adding on to that, the absence of any real reliable benchmark for legal, what advice do you have for law firms when it comes to comparing the relative aptitude or capability of AI models for different tasks in law? |
00:43:30 | AA: | Two suggestions. None of them is good. One is, build your own benchmark and because it’s so difficult, you’ve got to probably find partners, get united with other law firms and get together and there are papers on creating proper benchmarks. There is process to help you out to figure out how you’re supposed to do it and once you have that, that would be a huge help with any new model to figure out whether you want to even give this model a try and waste your time or your benchmark is telling you that it’s garbage and you don’t want to try it. So that’s the preferable option, but it takes time. The other is, there are benchmark websites that don’t do the benchmarking with pre-written questions. You as a human go on the website and there are two models which you don’t know which models these are and you have this question, legal question or whatever, you copy it there and it gives you two answers with each one of the the AI models and then all you have to do there is to say which one was better, and you click and then adds up all those numbers based on how people voted and then you can see the charts. And the charts are getting better, the leaderboard is getting better in terms of sometimes it has sections for comprehension, like which model is the best for comprehension, which model is best for knowledge and I think that’s the most reliable, I would say at the moment, there are multiple other benchmarks that have developed that the questions change, the numbers change, they fix many of the problems, but none of them, as far as I know, is specifically for law. So, you might use those online benchmarks that people vote on, and I do say that they are much more reliable because they’re using everyday real life things. It’s not like “a bunch of apples are on the ground and oranges and what do you want to do with them?” And you’ve got to eventually, if that doesn’t satisfy anyone, go and make your own benchmark because this story is not going to end here. They’re going to be newer and newer models and every time you want to figure out which one can do this specific task, which one cannot. |
00:45:21 | DT: | I guess this is the challenge that even nearly two years on from the release of ChatGPT and so many enterprise applications powered by generative AI in the market, the models are being released so quickly and use cases are being devised for them so quickly that we’re still really in a experimentation mode, aren’t we? We still have to, I guess, design our own experiments to work out what will work for our organisations and our teams. |
00:45:48 | AA: | That’s true. At the same time, we are not spending much time on them. We’re just reading the headlines. We’re not going and trying it. And like at the very beginning, you said there was a report every second lawyer is using generative AI. I’ve talked with many people in practice, I do not believe a single one of those surveys that say, like, more than 50% of law firms. And I’m really sorry for the people who are hearing, but apparently there are many law firms just for advertisement, they are saying that we are using them or using generative AI, being defined as loosely that they say that because, yeah, in my experience, many are not even touching it or have used it once or twice and that was it. |
00:46:23 | DT: | Yeah, I would echo that sentiment. I think just from my own anecdotal experience speaking to lawyers, legal practice managers, if that stat is correct, then it’s a very light touch, toe in the water approach to using generative AI, like “I’ve asked ChatGPT to help me write an email” or something like that and not really to the level of experimentation that you described, like playing around with parameters and prompt engineering techniques, models, other applications powered by the same large language models. I think you’re right that there’s still a lot of experimentation to do beyond, yeah, asking a few questions on ChatGPT. |
00:47:02 | AA: | Yeah and to me, it’s not a new shiny product because let me give you this example. If you see your GP and you’re very sick and your GP is experienced person, but they are very busy as well and you have this illness and they don’t diagnose you properly or they misdiagnose you and the reason is because they’re seeing a lot of patients, they don’t have time, even like an hour or two hour a week to read the new research about the illnesses, to learn about the new issues people are coming with and with those illnesses. So you don’t want that type of GP. You want someone to be able to learn as the things are getting better and updated. Same with technology, whether it’s generative AI or any other technology. You want to have at least a small amount of time every week to just go and try to discover what is out there and how these things work and some of them actually really help you save time but you’ve got to give them a try. Otherwise, yeah, it would never happen and I think many law firms, the problem is they are so busy. There’s no time to investigate what is good and what is bad and there’s too much information about gen AI. I’ve seen like many people just constantly say things. You never go and try all the 20 prompts that they share on their LinkedIn. No one does that. Why are you even sharing that? So the pressure is high. People don’t have time. It requires really, an organised policy in the law firm to say, “OK, once a week, half an hour, everybody’s got to go and try this a little bit.” |
00:48:27 | DT: | Yeah, you’re absolutely right. There’s so much noise out there. Those LinkedIn instant experts with their libraries of prompts. It’s probably the most egregious, but it’s true. This is a skill that lawyers need to be developing, leaving aside what we’ve been talking about today, which is how you can use some of these tools to improve your own work, similar to your metaphor, there’s an important learning exercise in understanding what generative AI is capable of to better understand the legal issues and business issues that your clients will be coming to you about. |
00:48:56 | AA: | Yeah, totally, and as I said, there are so many things that you’ve got to discover. Like I’ve never tried for a grant application. It’s too complicated. And I tried it, and it was, I can’t say, fantastic. None of them is fantastic. I had to verify the output and change it. It was such a good starting point. And for grants I would miss the deadline, I managed to submit and I even got one of them. And I’m so much more confident in using it and I know there’s a risk – sometimes it doesn’t work properly – but it gave me a decent first draft and that’s the thing I’m asking everyone. Give it a try and see whether the first draft is good enough and worth your time to, you know, analyse and yeah, improve it, or it’s not – it’s completely garbage and you don’t want to try that again. |
00:49:33 | DT: | So I guess the takeaway from today’s interview, if there was sort of one thing we wanted to leave our listeners with, give it a try for the use cases that you think will make sense for your organisation. Don’t expect too much, expect a great starting point or first draft, but not a final product and verify the results. |
00:49:50 | AA: | Yeah, exactly and don’t think that the model is going to stay the same. Two years ago, the models were just crap. Even 12 months ago, compared to current models, they were way worse than that. So you are making yourself ready for a future with better models and easier for you to catch up and so right now, probably everybody feels like there’s just too much out there to catch up and no one, you know, has the time to just digest that much information. So just getting them to give it a try and yeah, verify the output. |
00:50:18 | DT: | Armin, thank you so much for joining me on Hearsay. |
00:50:20 | AA: | No worries. Pleasure. |
00:50:20 | DT: | As always, you’ve been listening to Hearsay the Legal Podcast. I’d like to thank my guest today, Armin Alimardani, for coming on the show. Now, if you want to learn more about how you can harness the power of AI in your law firm, check out our episode with Jack Newton, the CEO of leading global practice management software company, Clio. That episode is called ‘The Digital Assistant Paradigm: The Future of AI Digital Assistants in Legal Practice Management’ and it’s episode 111. Now, if you’re an Australian legal practitioner, you can claim one continuing professional development point for listening to this episode. Whether an activity entitles you to claim a CPD unit is self assessed, as you know, but we suggest this episode entitles you to claim a practice management and business skills point. For more information on claiming and tracking your points on Hearsay, please go to our website. Hearsay the Legal Podcast is brought to you by Lext Australia, a legal technology company that makes the law easier to access and easier to practise, including your CPD. I’d like to ask you a favour, listeners. If you like Hearsay the Legal Podcast, please leave us a Google review. It helps other listeners to find us, and that keeps us in business. Thanks for listening, and I’ll see you on the next episode of Hearsay. |
You must be a subscriber to access this content.